Banks make decisions in prospecting (acquiring customers), underwriting (lending to consumers and businesses) and customer management (collection, customer servicing, up-sells etc.) in a regular course of business and these decisions are driven by predicting customer behavior through predictive models. Sound model management processes with governance, speed-to-market and automation is critical bedrock for decision management stability.
In this blog, we will provide an overview of Corridor Platform(CP), a leading decision workflow governance and automation solution, its capabilities in the model management process. We highlight key challenges to the model management process in the current Covid-19 driven crisis and how CP can address some of those challenges.
Model Management in Banking
Model Management is a closed-loop process involving model development/calibration, model validation, deployment & model monitoring. The overall process requires close coordination among the model development, validation, production and monitoring teams at the bank with robust controls & well-defined interfaces between teams. Speed-to-market and strong governance are critical for banks to compete nimbly and flexibly in the marketplace.
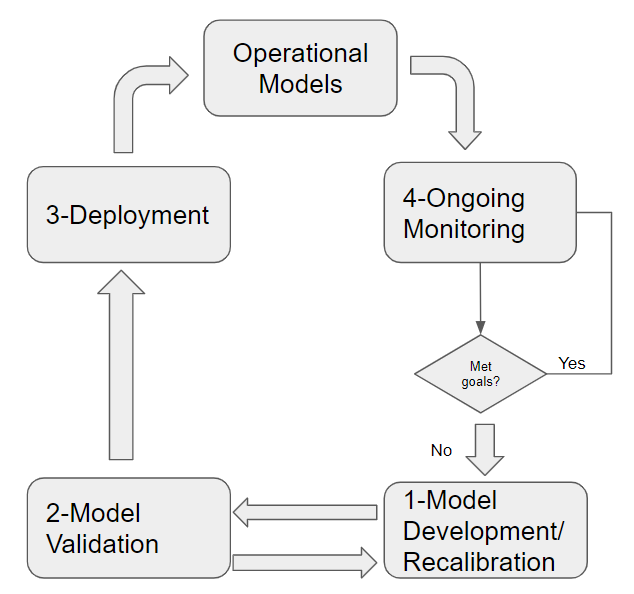
As an introduction, The Corridor team is mostly banking industry experts who have learned their skill underwriting and managing multi-billion dollar loan books through multiple recessionary cycles. We have taken our experience as end-users and incorporated our learnings in developing an integrated decision management workflow automation platform to help banks/regulated firms leapfrog legacy decisioning processes and technologies to a next gen capability/platform needed to win in the age of digitization.
Model Development
Authorized access to new data sources with controls: CP’s highly connected platforms allow rapid incorporation of new data sources with the right level of controls for authorized use, while providing complete flexibility for modelers to leverage AI for innovation in risk modeling while enhancing governance and traceability. In the example below, the account level forbearance data from the bureau is registered and governed for permissible use in models for underwriting with full lineage traceability of use in downstream models.
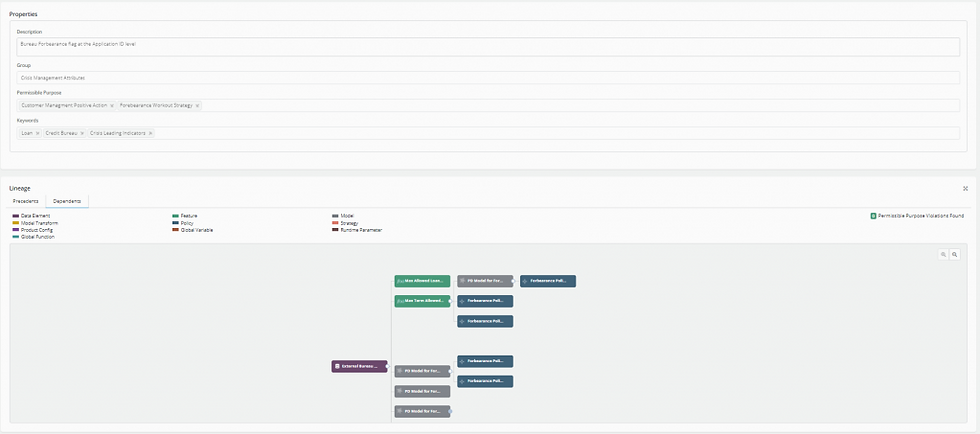
Integrate CP governed & connected data into the full cycle model development process:
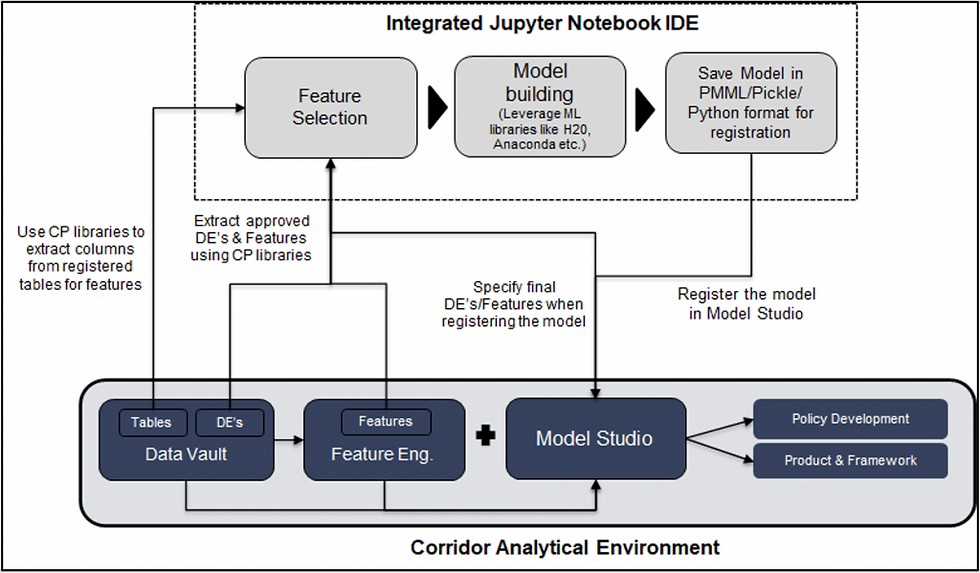
CP offers a rich library of routines that allow modelers to extract authorized data from CP to their modeling sandboxes though python and then have complete flexibility to use powerful ML/AI libraries like Anaconda, H2O, PyTorch to build robust models leveraging big data.
Rapidly develop and test new decision strategies using ‘English like’ rule writer: CP also supports rapid development of strategy overlay rules on model output using a strategy writer engine that is highly intuitive and easy for a policy writer to use. Strategy rules can be defined segment-wise or encompass all segments. In the example below, the score cutoff for default for high affected states by Covid-19 is tightened versus BAU, with an intuitive rule overlay on existing model scores.
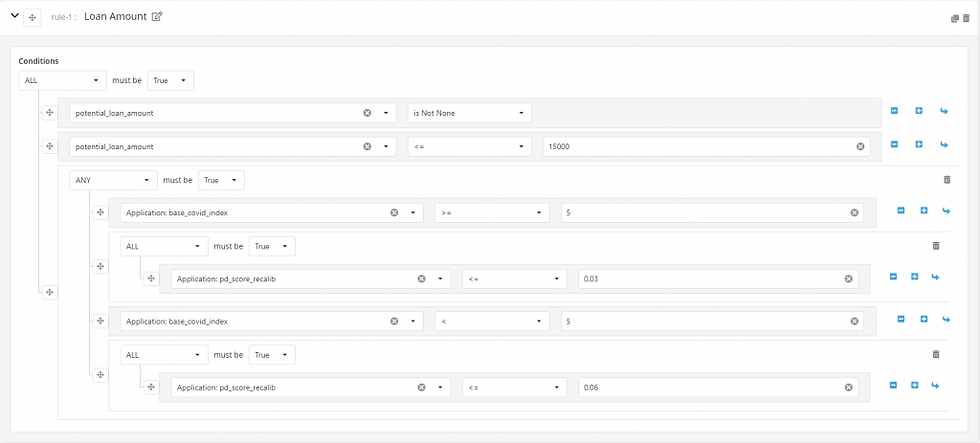
Model Validation
Highly configurable model validation module with workflow automation to support efficient interaction:
CP’s model validation module comes in two variants,
Model Validation – Single model, multiple validation datasets
Model Comparison – Multiple models, single validation datasets (ex: champion challenger scenarios)
The platform enables the model developer and reviewer to rapidly develop multiple scenarios for model validation (including extensive data segmentation), automate the development of standardized model performance metrics (ex: AUC curve, KS Tables etc.) and track the approval workflow process including multiple approval levels & audit trails through the platform. In the example below, the segmented validation for low FICO applicant base is lower than the benchmark and suggests that the modeler might need to put in some business rule overlays to address the issue. In addition, the following image shows the approval workflows audit engine which supports layered approvals with audit trails.
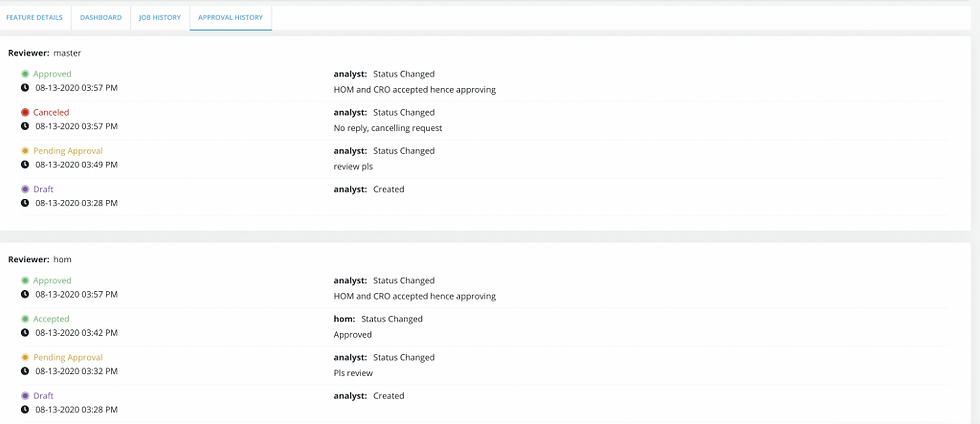
Moving Models from analytics to production & feedback loop
Decision artifact generation: CP automatically stitches together a standalone artifact that can be deployed seamlessly in the bank’s production systems. The artifact can score in batch (on Spark cluster) or real-time mode (on python) and exactly mirrors the logic developed during the analytic build process.
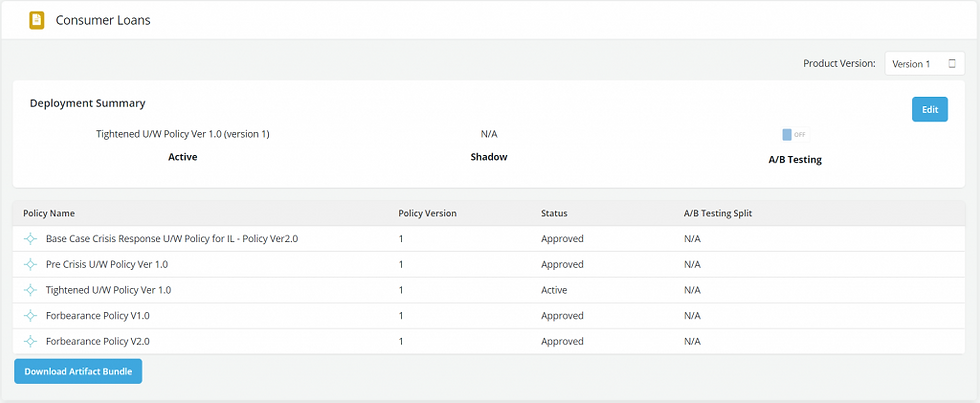
Enable rapid test-learn: CP offers automated workflows to measure impact of decisions based on models by allowing new performance data to be easily loaded and used for the measurement metrics which are standardized across model or product families.
Model Performance Monitoring
Automated model monitoring: CP provides capabilities for banks to easily set up automated performance monitoring of models at pre-scheduled periods as well as define performance benchmark thresholds, which if breached, resulting in an exception trigger report to the model monitoring team. This automates the monitoring process and facilitates management by exception. In the example below 16 iterations for model tracking on a weekly basis is setup with floor and ceiling AUC (Area Under Curve) of 0.55 & 0.85 respectively.
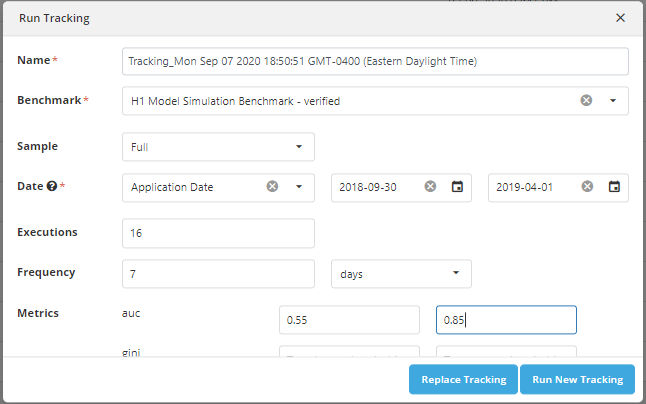
Segmental performance monitoring: CP allows the bank to easily setup and measure the segmental performance of BAU models to identify potential model ‘breaks’. In the example below, the model performance is unstable for the severe Covid-19 affected segment of customers by region (COVID severity cutoffs are based on COVID index defined at regional level).
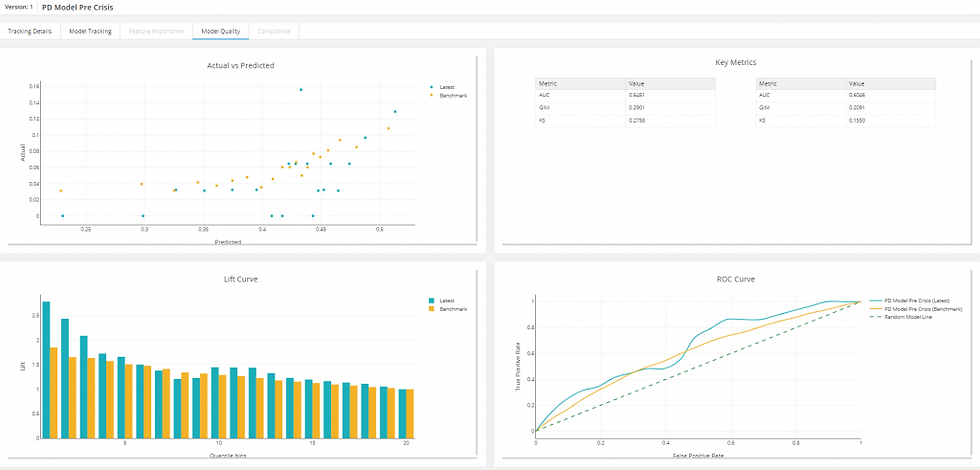
Ramifications of multiple waves of Covid-19 for Banks’ models
We are going through an unprecedented period of uncertainty based on multiple waves of the Covid-19 driven crisis and its economic impact on business sectors, geographic regions and customer behavior. From the chart below, it’s clear that multiple waves of Covid-19 driven crisis will result in uneven impact on different sectors and shape customer behavior in unique new ways.
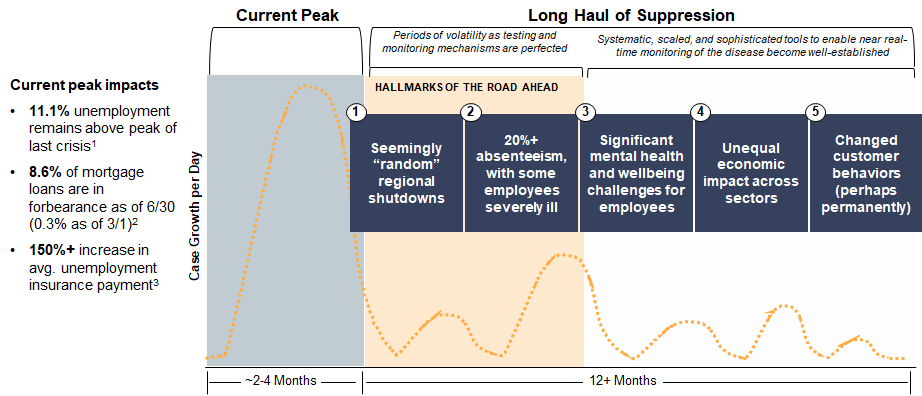
Source: Oliver Wyman insights
The Fed recently carried out a sensitivity analysis to assess the threat to banking resulting from the adverse effects of the pandemic. Though all participating banks look sufficiently capitalized for now, 25% of them could report CET1 capital ratio close to the regulatory minimum of 4.5% if the economy witnessed a W shaped recovery trajectory in upcoming quarters. This can be further compounded by Covid-19 waves.
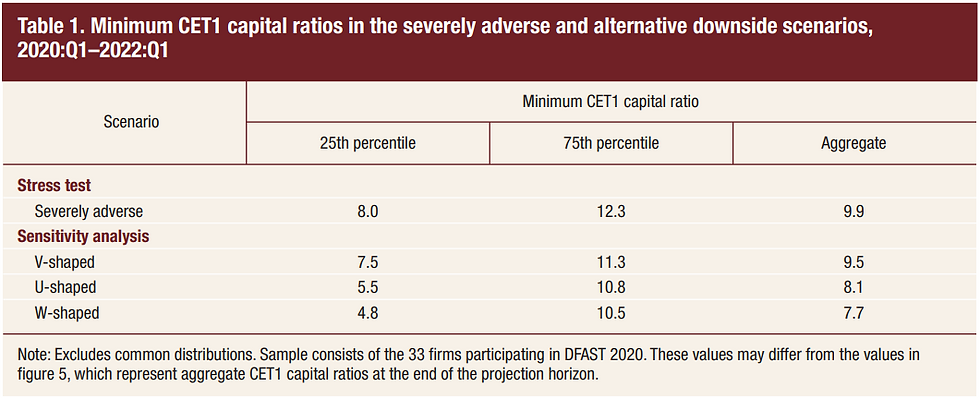
An obvious implication of the pandemic on banks’ models is that population characteristics have now changed in ways which were unimaginable for most experts at the time those models were built. Sectors like food services and air transport are expected to be impacted quite adversely across crisis scenarios based on analysis done by Oliver Wyman in its recently published report on model management (see an excerpt on industry analysis from the report below).
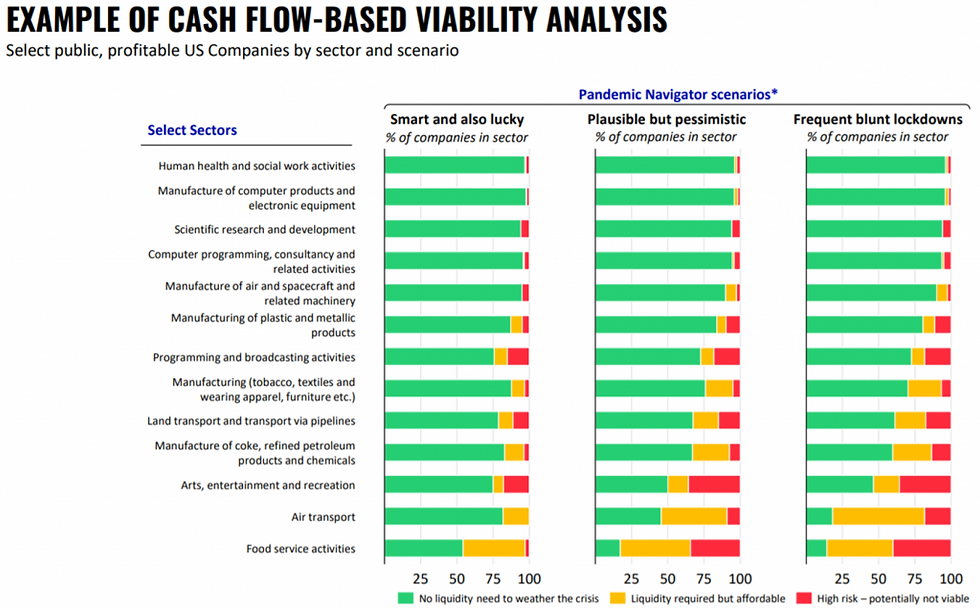
Source: Oliver Wyman – COVID-19 and Impact on the US Financial System July 2020
Strategic challenges for model management in retail credit and potential solutions leveraging Corridor’s decision management suite
Pain points in Model development
Relationships between predictors and outcome broken in crisis period: Due to the extreme and uncharted impact of Covid-19, traditional model relationships might break. For example, relationships between macroeconomic variables and default due to large scale deferrals. Banks have options for:
Retrain models using new data safely: Banks need the ability to bring in new data (ex: Bureau forbearances at loan level) with robust control & governance and safely incorporate that data for model retraining with right permissible use.
Build business overlay strategies for short term model fixes: Banks also need the ability to quickly update their decisions strategies (or business overlays) based on models. Due to lack of historical data or precedence in a highly uncertain environment, banks need the ability to use expert judgment and assumptions driven decision strategies instead of model retraining.
Flexibility in model development required due to lack of specific guidance in the pandemic period: Banks need the flexibility to develop multiple training datasets with exclusion/inclusion criteria's and segmental dataset which are approved for use by 1st/2nd line of defence teams and have strict governance protocols for model build and scenarios planning.
Corridor Solution
Corridor’s fully integrated solution facilitates necessary adjustments and recalibration of impacted ML models. ‘English like’ rule writer helps enhance models with expert judgement based overlays quickly (ex: stricter underwriting rules for worst-affected customer segments) that are essential in an uncertain crisis period with limited data availability for robust recalibration. Strong focus on governance & connectivity between model and strategies ensures that all there are no compliance violations (ex: fair lending requirements). The updated models and/or strategies can be quickly extracted as artifacts for production scoring.
Pain points in Model validation
More frequent model validation in the pandemic period which is still a highly manual process: Bank’s validation teams are working double time (so to say) to make sure they are able to test various validation scenarios (including segments) on BAU models and identify performance gaps.
Higher emphasis on ‘judgement calls’ in the validation process: We believe validation teams will now go beyond the quantitative assessment of model performance and have a qualitative assessment on strategy rule overlays required as compensating controls for model performance where recalibration might not be feasible in the short term.
Corridor Solution
With model validation becoming more recurrent during crisis, CP’s comparison and validation capabilities can be leveraged to validate models and overlays quickly. Validation teams can leverage standardized performance dashboards which are preapproved by the risk line of defence and generate all reports and metrics in one place. Automated approval workflows and automated model documentation supports rapid approval cycles and allows validation teams to manage large workloads of model validation in the current crisis.
Pain points in moving Models from analytics to production & feedback loop
Elongated time-to-market for production: Banks struggle to move analytics to production quickly with the need to recode the model and rule overlays in production, quality checks & extensive testing requirements before deploying into production and deriving benefits.
Highly manual test-learn feedback loop: Bank’s process to ‘test-learn’ once the analytics have been put to production is also time-consuming and mostly manual.
Corridor Solution
Corridor generates deployable artifacts in a single click. This enables the risk team to spend more time on designing and investigating model and strategy adjustments required as a result of crisis. Moving models from analytics to production becomes trivial in CP. As a feedback loop, performance data from these adjustments can be uploaded on the platform to assess if initial interventions need further refinement and the iteration cycle continues.
Pain points in Model performance monitoring
Segmental performance: Given the extreme swings in macroeconomic variables which were not available in development data during model build, model performance varies significantly by segments (ex: by Covid-19 regional indicators or employment sectors)
One-off crisis response actions: Massive one-time remedial measures like mass forbearances and federal stimulus checks have skewed the impact of timing and severity of default of retail loans. Models need to be monitored for degradation of performance and business overlays (or strategy rules) need to be actioned as compensating controls.
Performance management by exception: With the large number of models to monitor across products, customer segments and regions, banks model management teams are short-staffed to manage an essentially manual process.
Corridor Solution
CP’s scheduling capability structures and automates continuous monitoring of models impacted due to crisis. Monitoring team would be alerted when the model performance breaches performance thresholds. Model monitoring is automated with the ability to define schedules for iteration counts and time periods for monitoring. CP also supports segmental performance monitoring (ex. product segment, customer segment) to identify model ‘gaps’ (ex: model performance of a default model for high covid intensity states).
By addressing these pain points, we believe banks can develop resilient model management to manage the current crisis and overall better prepare to handle crises in the future. Banks also have a great opportunity to upgrade their decision management technology and thereby build a sound model management process to develop a sustainable differentiation in the marketplace.
Comments